Artificial Intelligence has been a game changer in numerous fields, from healthcare to retail to entertainment and art. Yet, new research suggests that we might have reached a tipping point: AI learning from AI-generated content.
This AI ouroboros—a serpent eating its own tail—could end quite badly. A research group from different universities in the UK has issued a warning about what they called "model collapse," a degenerative process that could entirely separate AI from reality.
In a paper titled "The Curse of Recursion: Training on Generated Data Makes Models Forget," researchers from Cambridge and Oxford universities, the University of Toronto, and Imperial College in London explain that model collapse occurs when "generated data ends up polluting the training set of the next generation of models.”\
“Being trained on polluted data, they then mis-perceive reality," they wrote.
In other words, the widespread content generated by AI being published online could be sucked back into AI systems, leading to distortions and inaccuracies.
This problem has been found in a range of learned generative models and tools, including Large Language Models (LLMs), Variational Autoencoders, and Gaussian Mixture Models. Over time, models begin to "forget the true underlying data distribution," leading to inaccurate representations of reality because the original information becomes so distorted that it stops resembling real-world data.
There are already instances where machine learning models are trained on AI-generated data. For instance, Language Learning Models (LLMs) are being intentionally trained on outputs from GPT-4. Similarly, DeviantArt, the online platform for artists, allows AI-created artwork to be published and used as training data for newer AI models.
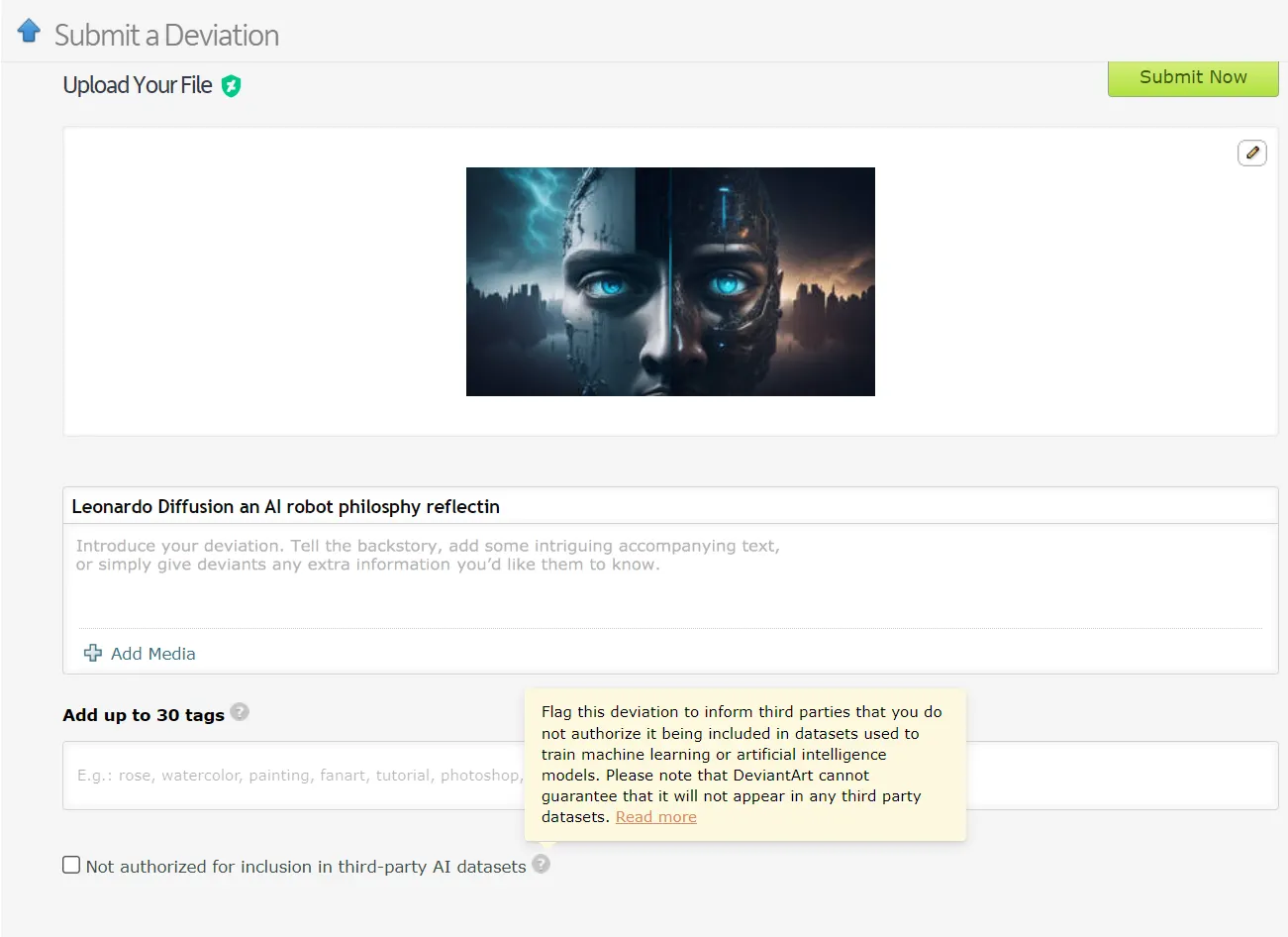
Much like attempting to indefinitely copy or clone something, these practices, according to the researchers, could lead to more instances of model collapse.
Given the serious implications of model collapse, access to the original data distribution is critical. AI models need real, human-produced data to accurately understand and simulate our world.
How To Prevent Model Collapse
There are two main causes for model collapse, according to the research paper. The primary one is "statistical approximation error," which is tied to the finite number of data samples. The secondary one is "functional approximation error," which stems from the margin of error used during the AI training not being properly configured. These errors can compound over generations, causing a cascading effect of worsening inaccuracies.
The paper articulates a “first-mover advantage” for training AI models. If we can maintain access to the original human-generated data source, we might prevent a detrimental distribution shift, and thus, model collapse.
Distinguishing AI-generated content at scale is a daunting challenge, however, which may require community-wide coordination.
Ultimately, the importance of data integrity and the influence of human information on AI is only as good as the data from which it, and the explosion in AI-generated content might end up being a double-edged sword for the industry. It’s “garbage in, garbage out”—AI based on AI content will lead to a lot of very smart, but “delusional,” machines.
How’s that for an ironic plot twist? Our machine offspring, learning more from each other than from us, become “delusional.” Next we’ll have to deal with a delusional, adolescent ChatGPT.