It was a corporate espionage story even a real human screenwriter couldn’t have dreamed up. OpenAI, which sparked the global obsession with AI last year, found itself in the headlines with the sudden dismissal and eventual reinstatement of Sam Altman, the company's CEO.
Even with Altman back where he started, a swirling cloud of questions remains, including what happened behind the scenes.
Some described the chaos as a HBO-level “Succession” or “Game of Thrones” battle. Others speculated it was because Altman shifted his focus to other companies like Worldcoin.
But the latest, and most compelling, theory says he was fired because of a single letter: Q.
Unnamed sources told Reuters that OpenAI CTO Mira Murati said that a major discovery—described as “Q Star” or “Q*”—was the impetus for the move against Altman, which was executed without participation from board chairman Greg Brockman, who subsequently resigned from OpenAI in protest.
What in the world is “Q*” and why should we care? It’s all about the most likely paths that AI development might take from here.
Unveiling the mystery of Q*
The enigmatic Q* cited by OpenAI's CTO Mira Murati has led to rampant speculation in the AI community. This term could refer to one of two distinct theories: Q-learning or the Q* algorithm from the Maryland Refutation Proof Procedure System (MRPPS). Understanding the difference between these two is crucial in grasping the potential impact of Q*.
Theory 1: Q-Learning
Q-learning is a type of reinforcement learning, a method where AI learns to make decisions by trial and error. In Q-learning, an agent learns to make decisions by estimating the “quality” of action-state combinations.
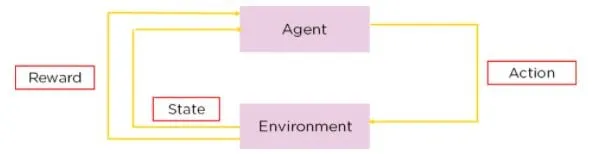
The difference between this approach and OpenAI’s current approach—known as Reinforcement Learning Through Human Feedback or RLHF—is that it does not rely on human interaction and does everything on its own.
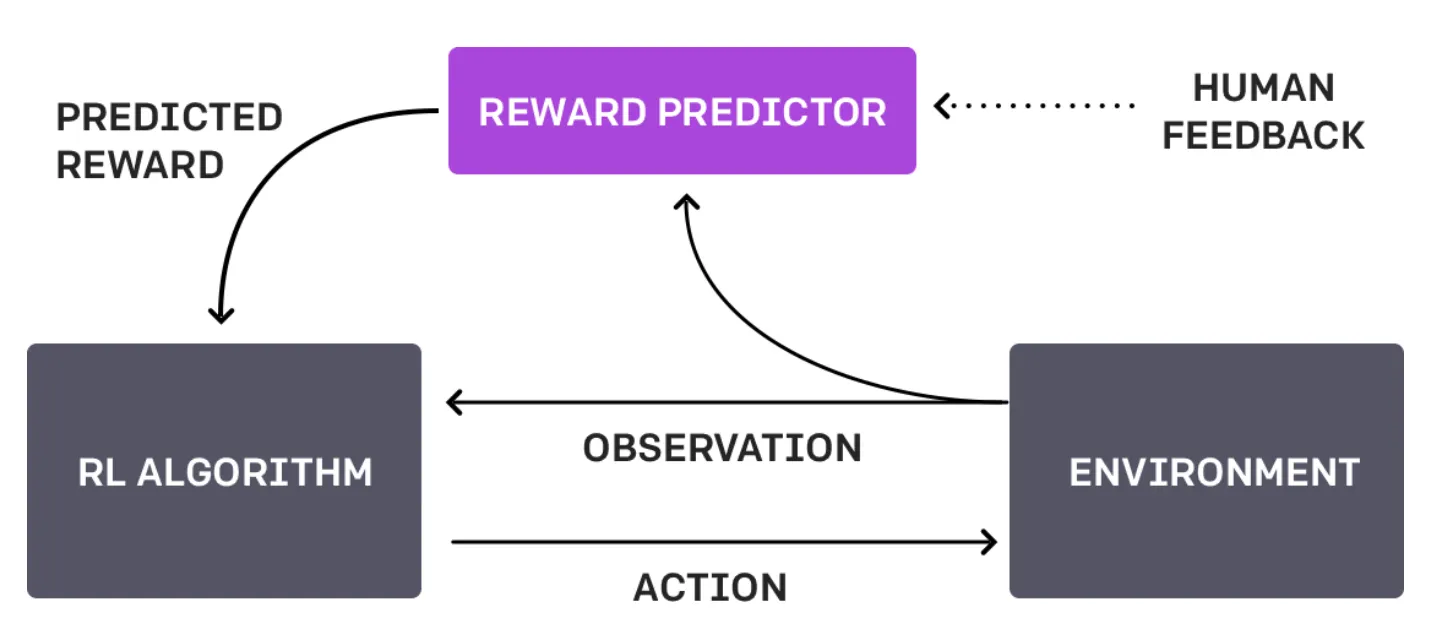
Imagine a robot navigating a maze. With Q-learning, it learns to find the quickest path to the exit by trying different routes, receiving positive rewards set by its own design when it moves closer to the exit and negative rewards when it hits a dead end. Over time, through trial and error, the robot develops a strategy (a "Q-table") that tells it the best action to take from each position in the maze. This process is autonomous, relying on the robot's interactions with its environment.
If the robot used RLHF, instead of finding things out on its own, a human might intervene when the robot reaches a junction to indicate whether the robot’s choice was wise or not.
This feedback could be in the form of direct commands ("turn left"), suggestions ("try the path with more light"), or evaluations of the robot's choices ("good robot" or "bad robot")
In Q-learning, Q* represents the desired state in which an agent knows exactly the best action to take in every state to maximize its total expected reward over time. In math terms, it satisfies the Bellman Equation.

Back in May, OpenAI published an article saying they "trained a model to achieve a new state-of-the-art in mathematical problem solving by rewarding each correct step of reasoning instead of simply rewarding the correct final answer." If they used Q-learning or a similar method to achieve this, that would unlock a whole new set of problems and situations that ChatGPT would be able to resolve natively.
Theory 2: Q* Algorithm from MRPPS
The Q* algorithm is a part of the Maryland Refutation Proof Procedure System (MRPPS). It's a sophisticated method for theorem-proving in AI, particularly in question-answering systems.
“The Q∗ algorithm generates nodes in the search space, applying semantic and syntactic information to direct the search. Semantics permits paths to be terminated and fruitful paths to be explored,” the research paper reads.
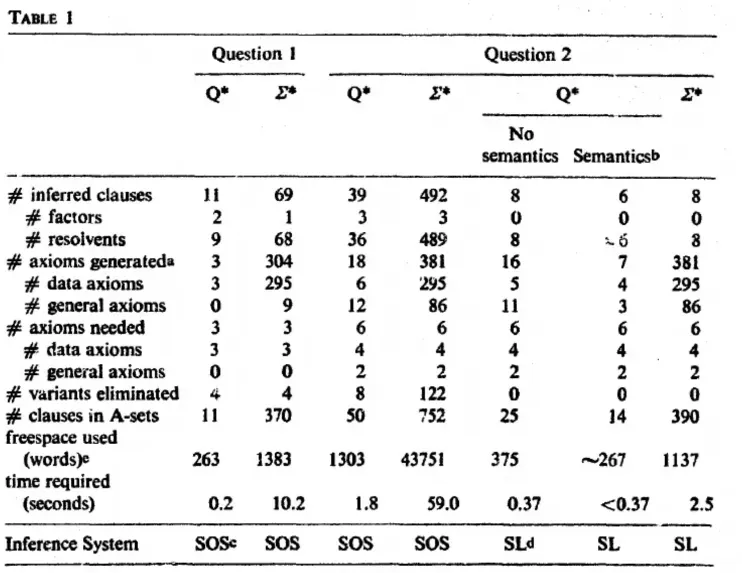
One way to explain the process is to consider the fictitious detective Sherlock Holmes trying to solve a complex case. He gathers clues (semantic information) and connects them logically (syntactic information) to reach a conclusion. The Q* algorithm works similarly in AI, combining semantic and syntactic information to navigate complex problem-solving processes.
This would imply that OpenAI is one step closer to having a model capable of understanding its reality beyond mere text prompts and more in line with the fictional J.A.R.V.I.S (for GenZers) or the Bat Computer (for boomers).
So, while Q-learning is about teaching AI to learn from interaction with its environment, the Q algorithm is more about improving AI's deductive capabilities. Understanding these distinctions is key to appreciating the potential implications OpenAI's “Q.” Both hold immense potential in advancing AI, but their applications and implications vary significantly.
All of this is just speculation, of course, as OpenAI has not explained the concept or even confirmed or denied the rumors that Q*—whatever it is—actually exists.
Potential implications of 'Q'*
OpenAI's rumored 'Q*' could have a vast and varied impact. If it's an advanced form of Q-learning, this could signify a leap in AI's ability to learn and adapt autonomously in complex environments, resolving a whole new set of problems. Such an advancement could enhance AI applications in areas like autonomous vehicles, where split-second decision-making based on ever-changing conditions is crucial.
On the other hand, if 'Q' relates to the Q algorithm from MRPPS, it could mark a significant step forward in AI's deductive reasoning and problem-solving capabilities. This would be particularly impactful in fields requiring deep analytical thinking, such as legal analysis, complex data interpretation, and even medical diagnosis.
Regardless of its exact nature, 'Q*' potentially represents a significant stride in AI development, so the fact that it’s at the core of an existential debate of OpenAI rings true. It could bring us closer to AI systems that are more intuitive, efficient, and capable of handling tasks that currently require high levels of human expertise. However, with such advancements come questions and concerns about AI ethics, safety, and the implications of increasingly powerful AI systems in our daily lives and society at large.
The good and the bad of Q*
Potential Benefits of Q*:
Enhanced Problem-Solving and Efficiency: If Q* is an advanced form of Q-learning or the Q* algorithm, it could lead to AI systems that solve complex problems more efficiently, benefiting sectors like healthcare, finance, and environmental management.
Better Human-AI Collaboration: An AI with improved learning or deductive capabilities could augment human work, leading to more effective collaboration in research, innovation, and daily tasks.
Advancements in Automation: 'Q*' could lead to more sophisticated automation technologies, improving productivity and potentially creating new industries and job opportunities.
Risks and Concerns:
Ethical and Safety Issues: As AI systems become more advanced, ensuring they operate ethically and safely becomes increasingly challenging. There's a risk of unintended consequences, especially if AI actions are not perfectly aligned with human values.
Privacy and Security: With more advanced AI, privacy and data security concerns escalate. AI systems capable of deeper understanding and interaction with data could be misused. So, imagine an AI that calls your romantic partner when you are cheating on them because it knows cheating is bad.
Economic Impacts: Increased automation and AI capabilities might lead to job displacement in certain sectors, necessitating societal adjustments and new approaches to workforce development. If an AI can do almost everything, why have human workers?
AI Misalignment: The risk that AI systems might develop goals or methods of operation that are misaligned with human intentions or welfare, potentially leading to harmful outcomes. Imagine a house-cleaning robot that's obsessed with tidiness and keeps throwing out your important papers? Or eliminates the creators of messes entirely?
The myth of AGI
Where does OpenAI's rumored Q* stand amidst the pursuit of Artificial General Intelligence (AGI) – the holy grail of AI research?
AGI refers to a machine's ability to understand, learn, and apply intelligence across various tasks, akin to human cognitive abilities. It's a form of AI that can generalize learning from one domain to another, demonstrating true adaptability and versatility.
Regardless of whether Q is an advanced form of Q-learning or relates to the Q algorithm, it's essential to understand that this doesn't equal achieving AGI. While 'Q*' might represent a significant step forward in specific AI capabilities, AGI encompasses a broader range of skills and understanding.
Achieving AGI would mean developing an AI that can perform any intellectual task that a human being can—an elusive milestone.
A machine that has achieved Q is not aware of its own existence and cannot yet reason beyond the boundaries of its pre-training data and human-set algorithms. So no, despite the buzz, “Q”is not quite the harbinger of our AI overlords just yet; it's more like a smart toaster that's learned to butter its own bread.
As for AGI ushering in the end of civilization, we might be overestimating our importance in the cosmic pecking order. OpenAI's Q* might be a step closer to the AI of our dreams (or nightmares), but it's not quite the AGI that'll ponder the meaning of life or its own silicon existence.
Remember, this is the same OpenAI that's been cautiously eyeing its ChatGPT like a parent watching a toddler with a marker—proud, but perpetually worried it'll draw on the walls of humanity. While “Q*” is a leap, AGI remains another bound away, and humanity's wall is safe for now.
Edited by Ryan Ozawa.