The lucrative world of proof-of-work altcoin mining was a spectacle to behold, rewarding miners with staggering profits... until Ethereum took a detour towards proof of stake. But an emerging industry is grabbing the attention of those who found themselves with hundreds of idle graphics cards after the merge: AI.
This newfound tech darling, spurred by the inception of ChatGPT, requires the computation of vast quantities of data, akin to Ethereum mining, and the best way to do such heavy lifting is with GPUs—lots of them.
However, this might not be the panacea many had hoped for because GPUs, the trusty workhorses of cryptocurrency mining, aren't exactly the MVPs in the AI training arena. Why? Let's distill this complex issue to its essence.
A tale of two needs
Here's the crux: Crypto mining and AI training have divergent appetites for their perfect GPUs. It's like dating two people with wildly different tastes. Proof-of-work cryptocurrencies have a thing for cards with tons of hash power, but couldn't care less about vRAM (Video Random Access Memory). In contrast, AI is a sucker for cards with abundant vRAM and gives a cold shoulder to hash power.
Picture hash power as the muscle of the operation—it's all about the number of computations your GPU can crunch per second. The higher the hash rate, the better your odds of unlocking that elusive block and basking in the crypto fortune. It's like a speed dating scenario: the more people you meet (calculations you make), the higher your chances of discovering a match (mining a block).
Conversely, vRAM is the capacity for handling and storing vast volumes of data simultaneously. It's the difference between juggling two balls or twenty. AI training is a notorious data glutton, demanding GPUs to handle and process colossal amounts of data concurrently. Using the dating example, going the vRAM route would be like inviting a crowd into a room and posing the same questions (data points) simultaneously to find the perfect match (AI model).
And here lies the paradox. Your buff, hash-power-loving GPU, perfect for mining Ethereum, is left gasping when it comes to training AI. It's like asking a world-class sprinter to compete in a marathon—it's just not their game.
A good backup plan?
Now, setting oversimplifications aside, crypto mining does indeed require high hash power, but it doesn't entirely sideline vRAM. Crypto mining utilizes vRAM as part of its mining algorithm, though the vRAM requirements are typically lower compared to those needed for AI training. For instance, a competent GPU for crypto mining might sport 4GB of vRAM, which is unsuitable to even run (not even think about train) an AI image generator like Stable Diffusion properly.
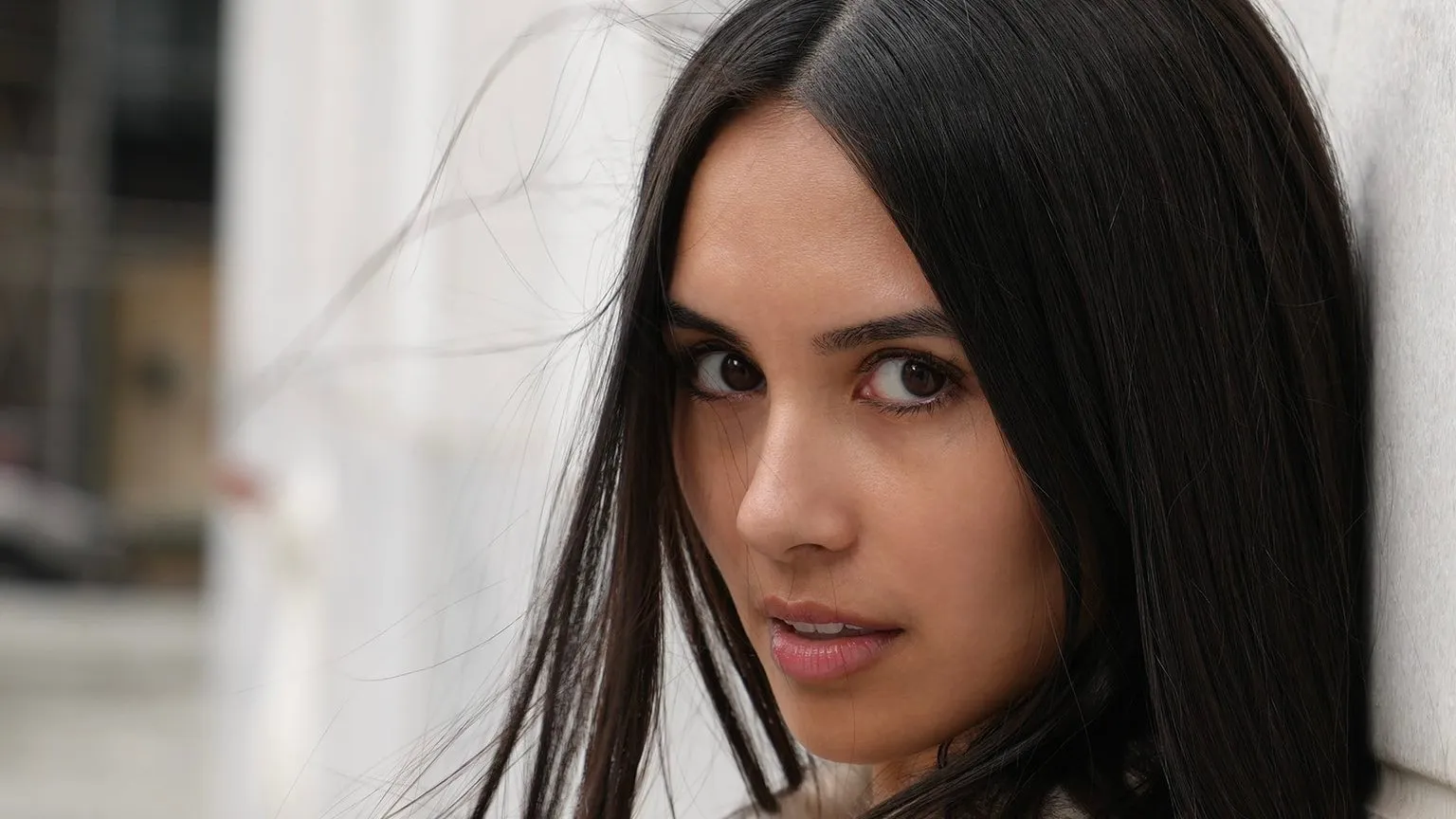
This 23-Year Old Snapchat Star Can Be Your AI Girlfriend—For a Price
Snapchat influencer Caryn Marjorie is harnessing the power of the AI revolution to fulfill the dreams of millions of her followers: becoming their girlfriend. She’s making some serious cash too. Marjorie, using OpenAI's GPT technology, has created CarynAI, an AI avatar that offers virtual companionship for a dollar per minute. It’s not a totally unfamiliar venture in the world of AI, but it’s yet another example of how the lines that separate the real from the unreal in AI are getting a little m...
To put things in context, one of the best GPUs for ETH mining was the Nvidia RTX 3060Ti with 60MH/s of hashpower and 8GB of vRAM. In contrast, OpenAI used Nvidia’s A100 and V100 models: A V100 comes with 32GB of vRAM and the A100 models handle up to 80GB.
But this doesn't imply that GPUs used for crypto mining are entirely redundant for AI training. GPUs with comparatively lower vRAM can still be employed for training smaller AI models or tasks that don't require significant vRAM.
“There are many AI operations and models that function quickly and efficiently on legacy cards,” Scott Norris, CEO and founder of Optiminer, told Decrypt, adding that entrepreneurs would have to properly study which AI application they focus on. GPUs used for crypto mining can work in “AI farms with either custom models or slightly adjusted models,” he said.
Norris mentioned that some crypto mining companies have already made the switch to AI operations. Omega AI is one example. Hive Blockchain and Hut8 Mining are also testing their luck.
So, while this solution may not be as lucrative as your good, old crypto mining, it could serve as a viable “plan C”—if your “plan B” of mining alternate proof-of-work altcoins seems bleak.
Interested in learning more about AI? Check out our latest Decrypt U course, “Getting Started with AI.” It covers everything from the history of AI to machine learning, ChatGPT, ChainGPT, and more. Find out more here.