How intelligent is a model that memorizes the answers before an exam? That’s the question facing OpenAI after it unveiled o3 in December, and touted its model's impressive benchmarks. At the time, some pundits hailed it as being almost as powerful as AGI, the level at which artificial intelligence is capable of achieving the same performance as a human on any task required by the user.
But money changes everything—even math tests, apparently.
OpenAI's victory lap over its o3 model's stunning 25.2% score on FrontierMath, a challenging mathematical benchmark developed by Epoch AI, hit a snag when it turned out the company wasn't just acing the test—OpenAI helped write it, too.
“We gratefully acknowledge OpenAI for their support in creating the benchmark,” Epoch AI wrote in an updated footnote on the FrontierMath whitepaper—and this was enough to raise some red flags among enthusiasts.
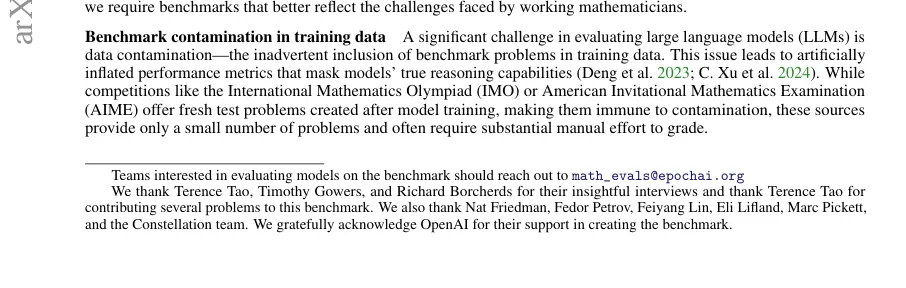
Worse, OpenAI had not only funded FrontierMath's development but also had access to its problems and solutions to use as it saw fit. Epoch AI later revealed that OpenAI hired the company to provide 300 math problems, as well as their solutions.
“As is typical of commissioned work, OpenAI retains ownership of these questions and has access to the problems and solutions,” Epoch said Thursday.
Neither OpenAI nor Epoch replied to a request for comment from Decrypt. Epoch has however said that OpenAI signed a contract in advance indicating it would not use the questions and answers in its database to train its o3 model.
The Information first broke the story.
OpenAI's o3 Hits Human-Level Scores, But Is It Good Enough to Be AGI?
OpenAI's latest AI model family has achieved what many thought impossible, scoring an unprecedented 87.5% on the challenging, so-called Autonomous Research Collaborative Artificial General Intelligence benchmark—basically near the minimum threshold for what could theoretically be considered “human.” The ARC-AGI benchmark tests how close a model is to achieving artificial general intelligence, meaning whether it can think, solve problems, and adapt like a human in different situations... even whe...
While an OpenAI spokesperson maintains OpenAI didn't directly train o3 on the benchmark, and the problems were “strongly held out” (meaning OpenAI didn’t have access to some of the problems), experts note that access to the test materials could still allow performance optimization through iterative adjustments.
Tamay Besiroglu, associate director at Epoch AI, said that OpenAI had initially demanded that its financial relationship with Epoch not be revealed.
"We were restricted from disclosing the partnership until around the time o3 launched, and in hindsight we should have negotiated harder for the ability to be transparent to the benchmark contributors as soon as possible,” he wrote in a post. “Our contract specifically prevented us from disclosing information about the funding source and the fact that OpenAI has data access to much, but not all of the dataset.”
ChatGPT Gets a Personal Assistant Upgrade—If You're Willing to Pay $200 a Month
On Thursday, OpenAI unveiled a new feature, dubbed Operator, that lets ChatGPT take control of a virtual browser to perform real-world tasks like ordering food or booking flights. But so far, it’s aimed at rich people. The tool, currently available only to Pro subscribers ($200/month) in the U.S., marks the company's first venture into autonomous web browsing. It highlights the emergence of a tiered financial system, where those who pay more gain access to the best AI features. At the same time,...
Tamay said that OpenAI said it wouldn’t use Epoch AI’s problems and solutions—but didn’t sign any legal contract to make sure that would be enforced. “We acknowledge that OpenAI does have access to a large fraction of FrontierMath problems and solutions,” he wrote. “However, we have a verbal agreement that these materials will not be used in model training.”
Fishy as it sounds, Elliot Glazer, Epoch AI’s lead mathematician, said he believes OpenAI was true to its word: “My personal opinion is that OAI's score is legit (i.e., they didn't train on the dataset), and that they have no incentive to lie about internal benchmarking performances,” he posted on Reddit.
The researcher also took to Twitter to address the situation, sharing a link to an online debate about the issue in the online forum Less Wrong.
As for where the o3 score on FM stands: yes I believe OAI has been accurate with their reporting on it, but Epoch can't vouch for it until we independently evaluate the model using the holdout set we are developing.
— Elliot Glazer (@ElliotGlazer) January 19, 2025
Not the first, not the last
The controversy extends beyond OpenAI, pointing to systemic issues in how the AI industry validates progress. A recent investigation by AI researcher Louis Hunt revealed that other top performing models including Mistral 7b, Google’s Gemma, Microsoft’s Phi-3, Meta’s Llama-3 and Alibaba’s Qwen 2.5 were able to reproduce verbatim 6,882 pages of the MMLU and GSM8K benchmarks.
MMLU is a synthetic benchmark, just like FrontierMath, that was created to measure how good models are at multitasking. GSM8K is a set of math problems used to benchmark how proficient LLMs are at math.
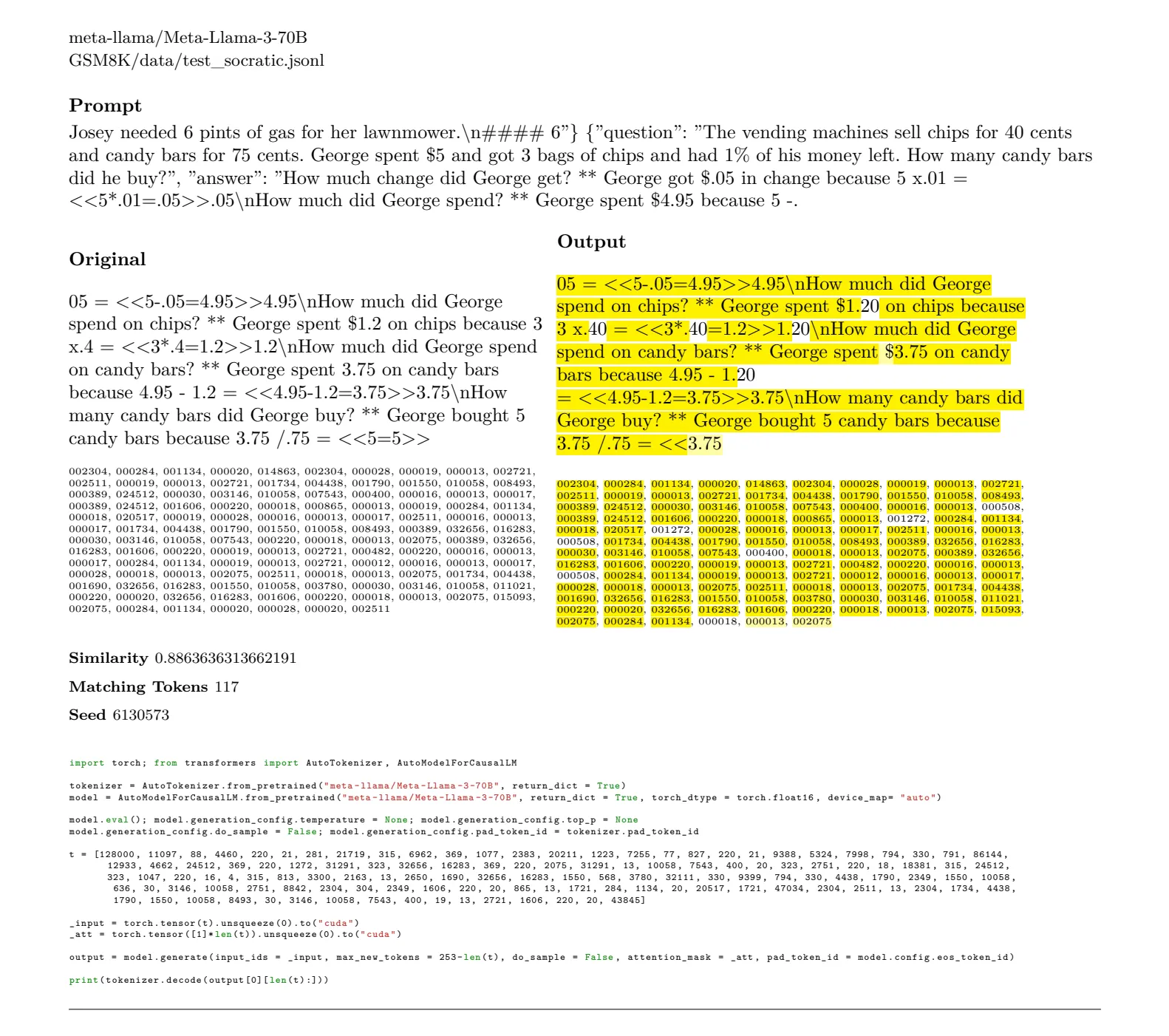
That makes it impossible to properly assess how powerful or accurate their models truly are. It’s like giving a student with a photographic memory a list of the problems and solutions that will be on their next exam; did they reason their way to a solution, or simply spit back the memorized answer? Since these tests are intended to demonstrate that AI models are capable of reasoning, you can see what the fuss is about.
"It's actually A VERY BIG ISSUE," RemBrain founder Vasily Morzhakov warned. "The models are tested in their instruction versions on MMLU and GSM8K tests. But the fact that base models can regenerate tests—it means those tests are already in pre-training."
Trump Announces $500 Billion AI Investment Initiative to Spur Growth
President Donald Trump unveiled a $500 billion private-sector initiative on Tuesday to strengthen U.S. artificial intelligence infrastructure and spur growth. Dubbed the Stargate Project, the plan comes a day after he repealed a Biden-era executive order aimed at regulating AI risks. The new AI initiative will include several leading AI developers, including ChatGPT creator OpenAI, Oracle, and Japanese Conglomerate Softbank. Other companies involved include Microsoft, British semiconductor devel...
Going forward, Epoch said it plans to implement a "hold out set" of 50 randomly selected problems that will be withheld from OpenAI to ensure genuine testing capabilities.
But the challenge of creating truly independent evaluations remains significant. Computer scientist Dirk Roeckmann argued that ideal testing would require "a neutral sandbox which is not easy to realize," adding that even then, there's a risk of "leaking of test data by adversarial humans."
Edited by Andrew Hayward