Artificial intelligence systems are being embedded with racist tendencies, causing machines to replicate human biases, experts warn. And as AI adoption soars, it could run the risk of perpetuating racial imbalance through a tool many believe will help advance civilization.
In a recently deleted BuzzFeed article, the author used AI image generation tool Midjourney to create depictions of Barbie dolls from different countries—and the results weren't well received. The German Barbie wore a Nazi SS uniform, the South Sudanese Barbie held a gun, and the Lebanese Barbie was posed in front of a rubbled building.
It’s a relatively lightweight example, but it points to potentially deeper and more consequential results as AI technology is wielded for an array of real-world use cases. And it isn't the first time AI has been called racist.
THEY GAVE SOUTH SUDAN BARBIE A GUN pic.twitter.com/buhOrzxjAc
— Wagatwe Wanjuki 🇰🇪 🇧🇸 (@wagatwe) July 8, 2023
In 2009, Nikon's face detection software would ask Asian people if they were blinking. In 2016, an AI tool used by U.S. courts to assess the likelihood that criminals would reoffend predicted twice as many false positives for black defendants (45%) than white offenders (23%), according to analysis from ProPublica.
More recently, Google's Vision Cloud misidentified dark-skinned individuals holding a handheld thermometer for a ”gun,” while light-skinned individuals were labeled as wielding an “electronic device.”
AI's tendency to display racial bias has caused the UK data watchdog Information Commissioner’s Office (ICO) to investigate the issue, claiming it could lead to "damaging consequences for people's lives."
"Tackling AI-driven discrimination is one of the ICO’s key priorities that we set out in our three-year strategic plan ICO25," a spokesperson told Decrypt. "Those working with AI must take care to mitigate these risks, especially where AI is being used to make decisions that can affect people’s lives."
You’re Not Imagining It: Top AI Chatbots Have Political Biases, Researchers Say
Popular AI chatbots have distinct political biases, according to tests performed by computer scientists and documented in a recent research paper. The scientists determined that OpenAI's ChatGPT and its new GPT-4 model to be the most left-leaning libertarian chatbot, while Meta's LLaMA leaned the furthest right and most authoritarian. "Our findings reveal that pretrained [language models] do have political leanings that reinforce the polarization present in pretraining corpora, propagating socia...
A recent study from infrastructure software company Progress highlighted that 78% of business and IT decision-makers believe data bias will become a bigger concern as AI and machine learning use increases, but only 13% are currently addressing it.
Earlier this month, researchers from the University of Washington, Carnegie Mellon University and Xi’an Jiaotong University found that generative AI tools also have different political biases, depending on where the tool’s corpus of data was collected and what information the tool was processing.
"There's nothing inherently racist about AI," Migüel Jetté, VP of AI at speech-to-text transcription company Rev, told Decrypt. "It's all in the process of us trying to understand how to build these things properly."
How does racial bias develop?
AI is trained on various datasets in order to develop their "intelligence." The dataset builds the AI model through a learning process, teaching it to act in a certain way. Unfortunately, this means that any biases entrenched within the dataset are mirrored and ultimately amplified by the final product.
For example, Rev's AI transcription service has been trained on millions of hours of voice data in order to translate audio entered by clients. If the original dataset excludes certain voices, accents, or groups, it'll have a much harder time being able to translate for those people.
"Dataset is the biggest reason these sorts of biases come in," Jetté explained. "What you show your algorithm and what you're telling the algorithm to learn—if that's not varied enough, then the algorithm won't be able to understand that stuff."
While the stakes are fairly low for Rev, where a limited dataset simply means not being able to translate certain accents, significantly worse outcomes can happen as AI seeps further into our daily lives.
For example, AI is already widely used in human resources, recruiting, and hiring, directly affecting economic outcomes of millions of people.
And by 2026, all new vehicles sold in the EU will require in-cabin monitoring that will detect driver drowsiness or distraction. If this AI system only works consistently with light-skinned people, then there could be a significantly higher possibility of a crash due to the system’s failure.
"In the field that we are focusing on—in-cabin monitoring for the automotive industry—if the system fails to detect whether the driver is drowsy or distracted, that might have life-critical implications," Richard Bremer, CEO of synthetic dataset company Devant, told Decrypt. "There are so many camera-based systems that are, step-by-step, entering different parts of our lives. We are not taking data seriously enough, in my opinion."
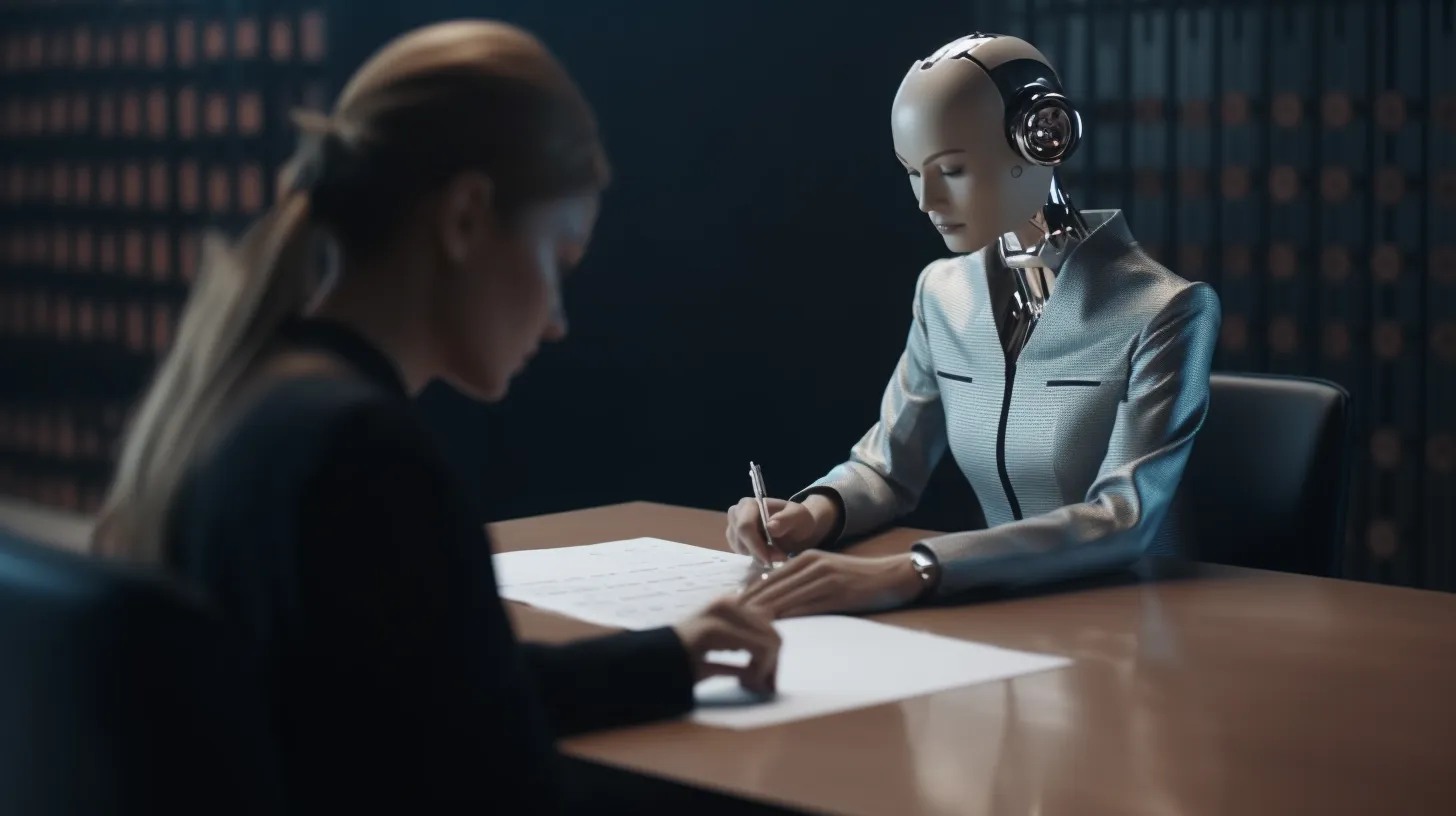
AI Has Most Americans Scared About Privacy, Job Losses
Americans feel apprehensive about the growing use of artificial intelligence (AI) in hiring and evaluating workers, according to a new study released by the Pew Research Center. Pew Research surveyed 11,004 U.S. adults in mid-December of 2022, asking participants for their views on AI's impact on the workforce. While some respondents acknowledged the efficiency of AI-driven recruitment, many expressed fears that the technology might invade privacy, impact evaluations, and lead to job losses. Acc...
Devant creates synthetic data sets of digital humans for camera-based AI applications, in order to battle biases that often occur in real-world datasets.
"If you focus on only real data, you will focus on gathering the data that is easily accessible. And the thing is that the data that is easily accessible is not always creating the best possible coverage of every possible real life scenario," Bremer explained. "The performance [of AI] is restricted to the data you have available. That's the problem that you face."
As a result, Devant supplies clients with large and diverse computer-generated datasets. Each image takes "just a few seconds" to generate using in-house automations, taking 3D content from Devant's large library of content.
However, having a representative dataset only goes so far—racial bias can still exist in the end product. For this reason the next step is bias testing, where the developers search for bias related performance issues.
"Testing for bias is a crucial aspect of bias mitigation, and I advocate for bias testing as a governance issue," Shingai Manjengwa, head of AI education at generative AI company ChainML, told Decrypt. “One has to assess each case individually. Even if a dataset is balanced, it can still carry bias."
There are a number of ways that a balanced dataset can result in biased results. There are algorithmic and model biases that can appear (i.e. linear regression has a bias towards linear relationships), as well as measurement and selection biases created in the source data.
"Interpretation or confirmation bias can also occur when analyzing model results." Manjengwa said. "This list of biases isn’t exhaustive. That’s why I advocate for bias testing as part of the machine learning process."
A diverse team
Diversity in a workplace plays an important role when it comes to testing an AI product.
"We can avoid some cases of bias when someone from a different background or race to everyone else on the team can highlight issues that an otherwise homogenous group of people would not see," Manjengwa told Decrypt. "It’s more than just their presence on the team. That team member must feel empowered to raise issues, and the team must be open to discussing and responding when concerns are raised.”
An example of this working within the industry is when Parinaz Sobhani, head of AI at Georgian, discovered that TurnItIn—a popular plagiarism detection tool used by universities—was biased against non-native English speakers.
The issue was only discovered due to having a non-English speaker on the team, and that resulted in a better, more inclusive product. This is a clear example of how diversity within the workforce can improve the efficiency of testing to prevent racial bias in AI.
Do AI Sex Robots Require Consent Too?
For most sex toys, the idea of asking for consent is laughable. But with the recent, meteoric rise of AI-powered tools, conversations around AI sex toys and consent have begun. And they’re getting serious. The sex robots on the market today aren't the most convincing human replicas (see: Emma), only conversing in basic language with frigid movements and unable to replace a real-life partner. Still, roughly 56,000 sex robots are sold every year, robot brothels are popping up across the world, and...
According to techUK, just 8.5% of senior leaders in UK tech are from ethnic minority groups. However, things are looking up for diversity in the AI industry, with a 2021 report showing that over half (54.4%) of AI PhD students in the United States were from ethnic minorities. That said, only a small number of students (2.4%) identified as Black or African American.
Organizations like Black in AI are working to bring this figure to a more representative number through workshops, events, and other initiatives. These advocates say diversity in AI isn't just a moral goal, but an important step to ensuring that AI systems work for everyone.
Unfortunately, even with a representative dataset, rigorous testing, and a diverse workplace, racial bias can still exist within AI models. Offensive results can particularly be an issue when AI is being used for unforeseen use cases.
"Generative AI is quite powerful and applicable to a lot of things," Jetté said. "People are kind of stretching the boundaries a little when they try these things. And then surprising things happen."
AI-Generated Books on Amazon Could Give Deadly Advice
The proliferation of AI-generated guidebooks sold on Amazon could have deadly consequences, experts warn. From cookbooks to travel guides, human authors are warning readers that artificial intelligence could lead them far astray. The latest cautionary tale about blindly trusting the advice of AI comes from the otherwise obscure world of mushroom hunting. The New York Mycological Society recently sounded the alarm on social media about the dangers posed by dubious foraging books believed to be cr...
Developers can only stress test their products so much—especially with seemingly limitless products like generative AI—that some mistakes are bound to slip through the cracks.
For this reason, AI users also carry part of the blame. Instead of using racist results for clicks online, users could report it to the dev team to help reduce the reproduction of such results within large language models (LLMs) in the future.
"The analogy I can offer is that we can and do regulate pedestrians (AI users) but more impactful gains can be had by requiring drivers licenses and car registration because of the damage vehicles (AI developers) can do," Manjengwa explained, "Addressing bias in AI is a multifaceted team sport that requires everyone—from users to practitioners to producers of LLMs—to participate and work towards fairer outcomes."